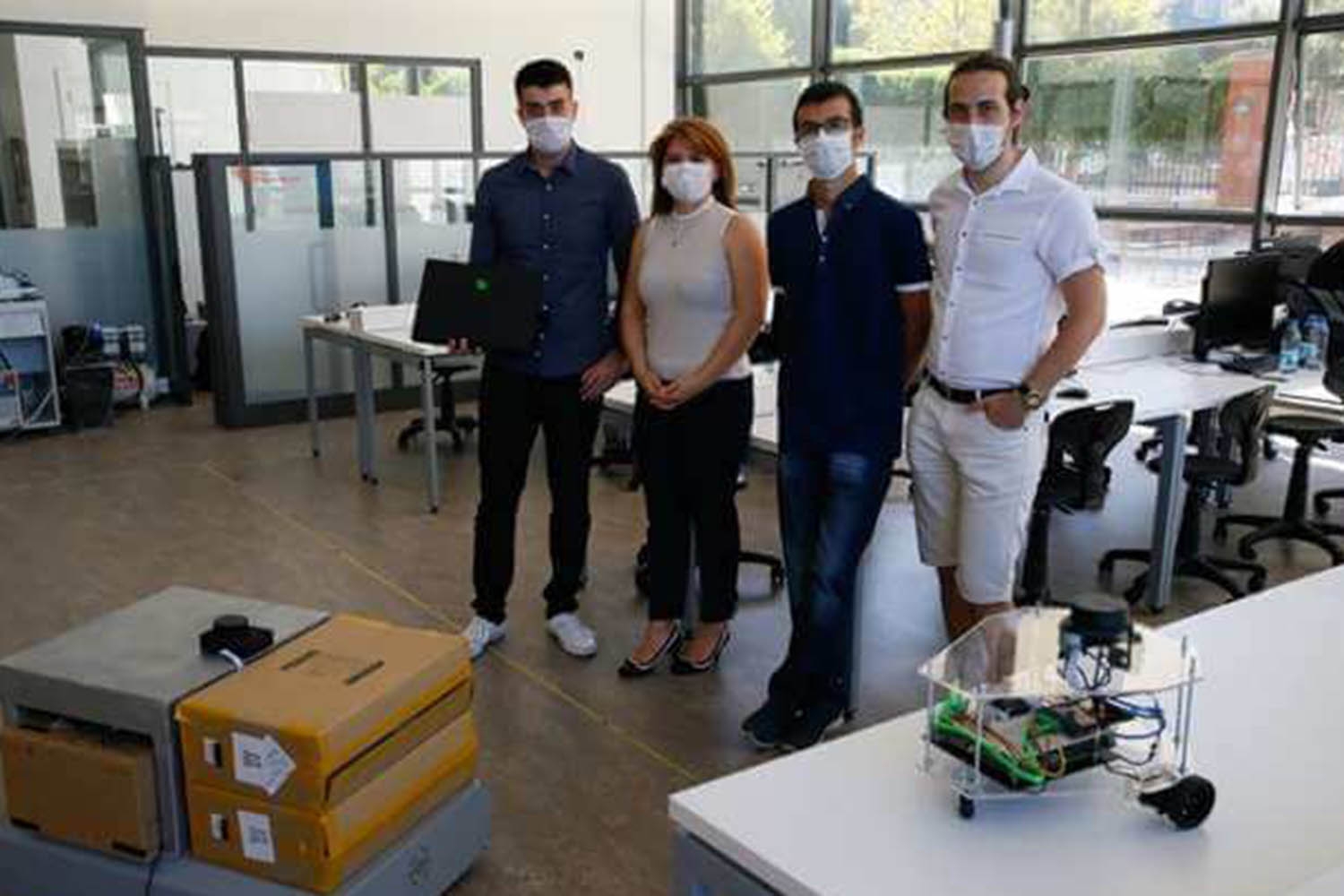
Self-routing autonomous robots by IUE engineers
An autonomous robot that can re-route using artificial intelligence, when it encounters an obstacle, has been developed with the project ...
Course Name |
Introduction to Neural Networks
|
Code
|
Semester
|
Theory
(hour/week) |
Application/Lab
(hour/week) |
Local Credits
|
ECTS
|
CE 470
|
Fall/Spring
|
3
|
0
|
3
|
5
|
Prerequisites |
None
|
|||||
Course Language |
English
|
|||||
Course Type |
Elective
|
|||||
Course Level |
First Cycle
|
|||||
Mode of Delivery | - | |||||
Teaching Methods and Techniques of the Course | Problem SolvingCase StudyLecture / Presentation | |||||
National Occupation Classification | - | |||||
Course Coordinator | ||||||
Course Lecturer(s) | - | |||||
Assistant(s) | - |
Course Objectives | This course will introduce the fundamental principles and algorithms of Artificial Neural Network (ANN) systems. The course will cover many subjects including basic neuron model, simple perceptron, adaptive linear element, Least Mean Square (LMS) algorithm, Multi Layer Perceptron (MLP), Back Propagation (BP) learning algorithm, Radial Basis Function (RBF) networks, Self Organizing Maps (SOM) and Learning Vector Quantization (LVQ), Support Vector Machines (SVMs), Continuous time and discrete time Hopfield networks, classification techniques, pattern recognition, signal processing and control applications. | |||||||||||||||||||||||||||||||||||||||||||||||||||||
Learning Outcomes |
|
|||||||||||||||||||||||||||||||||||||||||||||||||||||
Course Description | The following topics will be included in the course: The main neural network architectures and learning algorithms, perceptrons and the LMS algorithm, back propagation learning, radial basis function networks, support vector machines, Kohonen’s self organizing feature maps, Hopfield networks, artificial neural networks for signal processing, pattern recognition and control. |
|
Core Courses | |
Major Area Courses | ||
Supportive Courses | ||
Media and Management Skills Courses | ||
Transferable Skill Courses |
Week | Subjects | Related Preparation | Learning Outcome |
1 | Biological motivation. Historical remarks on artificial neural networks. Applications of artificial neural networks. A taxonomy of artificial neural network models and learning algorithms. | Introduction. S. Haykin, Neural Networks and Learning Machines, Pearson Education, 3rd Ed., 2009, ISBN13 9780131293762 ISBN10 0131293761. Lecture Notes. | |
2 | General artificial neuron model. Discretevalued perceptron model, threshold logic and their limitations. Discretetime (dynamical) Hopfield networks. Hebb’s rule. Connection wieght matrix as an outer product of memory patterns. | Chapter 1. S. Haykin, Neural Networks and Learning Machines, Pearson Education, 3rd Ed., 2009, ISBN13 9780131293762 ISBN10 0131293761. Lecture Notes. | |
3 | Supervised learning. Perceptron learning algorithm. Adaptive linear element. Supervised learning as output error minimization problem. Gradient descent algorithm for minimization. Least mean square rule. | Chapter 2. S. Haykin, Neural Networks and Learning Machines, Pearson Education, 3rd Ed., 2009, ISBN13 9780131293762 ISBN10 0131293761. Lecture Notes. | |
4 | Single layer, continuous valued perceptron. Nonlinear (sigmoidal) activation function. Delta rule. Batch mode and pattern mode gradient descent algorithms. Convergence conditions for deterministic and stochastic gradient descent algorithms. | Chapter 3. Chapter 4: Sections 4.1, 4.2, 4.16. S. Haykin, Neural Networks and Learning Machines, Pearson Education, 3rd Ed., 2009, ISBN13 9780131293762 ISBN10 0131293761. Lecture Notes. | |
5 | Multi layer perceptron as universal approximator. Function representation and approximation problems. Backpropagation Learning. Local minima problem. Overtraining. | Chapter 4: Sections 4.4, 4.5, 4.8, 4.10, 4.12. S. Haykin, Neural Networks and Learning Machines, Pearson Education, 3rd Ed., 2009, ISBN13 9780131293762 ISBN10 0131293761. Lecture Notes. | |
6 | Midterm Exam I. | ||
7 | Batch and pattern mode training. Training set versus test set. Overfitting problem. General practices for network training and testing. Signal processing and pattern recognition applications of multilayer perceptrons. | Chapter 4: Sections 4.3, 4.10., 4.11, 4.13, 4.14, 4.15, 4.19, 4.20. S. Haykin, Neural Networks and Learning Machines, Pearson Education, 3rd Ed., 2009, ISBN13 9780131293762 ISBN10 0131293761. | |
8 | Radial Basis Function (RBF) network. Backpropagation learning for determining linear weights, centers and widths parameters of RBF networks. Random selection of centers. Input versus input-output clustering for center and width determination. Regularization theory, mixture of Gaussian (conditional probability density function) model and neurofuzzy connections of RBF networks. | Chapter 5. S. Haykin, Neural Networks and Learning Machines, Pearson Education, 3rd Ed., 2009, ISBN13 9780131293762 ISBN10 0131293761 | |
9 | Parametric versus nonparametric methods for data representation. Unsupervised learning as a vector quantization problem. Competitive networks. Winner takes all networks. Kohonen’s self organizing feature map. Clustering. | Chapter 9. S. Haykin, Neural Networks and Learning Machines, Pearson Education, 3rd Ed., 2009, ISBN13 9780131293762 ISBN10 0131293761. | |
10 | Signal processing applications of artificial neural networks. Principal component analysis. Data compression and reduction. Image and 1D signal compression and transformation applications of artificial neural networks. | Chapter 8. S. Haykin, Neural Networks and Learning Machines, Pearson Education, 3rd Ed., 2009, ISBN13 9780131293762 ISBN10 0131293761 | |
11 | Midterm Exam II. | ||
12 | Pattern recognition applications of artificial neural networks. Artificial neural networks for feature extraction. Nonlinear feature mapping. Data fusion. Artificial neural networks as classifiers. Image and speech recognition applications. | Sections 1.4,1.5., 3.11, 4.7, 5.8, 6.7, S. Haykin, Neural Networks and Learning Machines, Pearson Education, 3rd Ed., 2009, ISBN13 9780131293762 ISBN10 0131293761. Lecture Notes. | |
13 | Implementation of artificial neural networks models and associated learning algorithms for signal processing, pattern recognition and control in MATLAB numerical software environment. | L. Fausett, Fundamentals of Neural Networks, Chapter 6, Prentice Hall, ISBN-13: 978-0133341867 | |
14 | Cumulative review of artificial neural networks models, learning algorithms and their applications. | S. Haykin, Neural Networks and Learning Machines, Pearson Education, 3rd Ed., 2009, ISBN13 9780131293762 ISBN10 0131293761. Lecture Notes. | |
15 | Semester Review | ||
16 | Final Exam |
Course Notes/Textbooks | S. Haykin, Neural Networks and Learning Machines, Pearson Education, 3rd Ed., 2009, ISBN13 9780131293762 ISBN10 0131293761 |
Suggested Readings/Materials | J. M. Zurada, Int. To Artificial Neural Systems, West Publishing Company, 1992 ISBN 053495460X, 9780534954604. L. Fausett, Fundamentals of Neural Networks, Prentice Hall, ISBN-13: 978-0133341867 |
Semester Activities | Number | Weigthing | LO 1 | LO 2 | LO 3 | LO 4 | LO 5 |
Participation | |||||||
Laboratory / Application | |||||||
Field Work | |||||||
Quizzes / Studio Critiques | |||||||
Portfolio | |||||||
Homework / Assignments |
5
|
20
|
|||||
Presentation / Jury | |||||||
Project |
1
|
30
|
|||||
Seminar / Workshop | |||||||
Oral Exams | |||||||
Midterm |
2
|
50
|
|||||
Final Exam | |||||||
Total |
Weighting of Semester Activities on the Final Grade |
100
|
|
Weighting of End-of-Semester Activities on the Final Grade | ||
Total |
Semester Activities | Number | Duration (Hours) | Workload |
---|---|---|---|
Theoretical Course Hours (Including exam week: 16 x total hours) |
16
|
3
|
48
|
Laboratory / Application Hours (Including exam week: '.16.' x total hours) |
16
|
0
|
|
Study Hours Out of Class |
14
|
3
|
42
|
Field Work |
0
|
||
Quizzes / Studio Critiques |
0
|
||
Portfolio |
0
|
||
Homework / Assignments |
2
|
3
|
6
|
Presentation / Jury |
0
|
||
Project |
1
|
24
|
24
|
Seminar / Workshop |
0
|
||
Oral Exam |
0
|
||
Midterms |
2
|
15
|
30
|
Final Exam |
0
|
||
Total |
150
|
#
|
PC Sub | Program Competencies/Outcomes |
* Contribution Level
|
||||
1
|
2
|
3
|
4
|
5
|
|||
1 |
To have knowledge in Mathematics, science, physics knowledge based on mathematics; mathematics with multiple variables, differential equations, statistics, optimization and linear algebra; to be able to use theoretical and applied knowledge in complex engineering problems |
-
|
-
|
-
|
-
|
-
|
|
2 |
To be able to identify, define, formulate, and solve complex mechatronics engineering problems; to be able to select and apply appropriate analysis and modeling methods for this purpose. |
-
|
-
|
-
|
-
|
-
|
|
3 |
To be able to design a complex electromechanical system, process, device or product with sensor, actuator, control, hardware, and software to meet specific requirements under realistic constraints and conditions; to be able to apply modern design methods for this purpose. |
-
|
-
|
-
|
-
|
-
|
|
4 |
To be able to develop, select and use modern techniques and tools necessary for the analysis and solution of complex problems encountered in Mechatronics Engineering applications; to be able to use information technologies effectively. |
-
|
-
|
-
|
-
|
-
|
|
5 |
To be able to design, conduct experiments, collect data, analyze and interpret results for investigating Mechatronics Engineering problems. |
-
|
-
|
-
|
-
|
-
|
|
6 |
To be able to work effectively in Mechatronics Engineering disciplinary and multidisciplinary teams; to be able to work individually. |
-
|
-
|
-
|
-
|
-
|
|
7 |
To be able to communicate effectively in Turkish, both in oral and written forms; to be able to author and comprehend written reports, to be able to prepare design and implementation reports, to present effectively, to be able to give and receive clear and comprehensible instructions. |
-
|
-
|
-
|
-
|
-
|
|
8 |
To have knowledge about global and social impact of engineering practices on health, environment, and safety; to have knowledge about contemporary issues as they pertain to engineering; to be aware of the legal ramifications of engineering solutions. |
-
|
-
|
-
|
-
|
-
|
|
9 |
To be aware of ethical behavior, professional and ethical responsibility; information on standards used in engineering applications. |
-
|
-
|
-
|
-
|
-
|
|
10 |
To have knowledge about industrial practices such as project management, risk management and change management; to have awareness of entrepreneurship and innovation; to have knowledge about sustainable development. |
-
|
-
|
-
|
-
|
-
|
|
11 |
Using a foreign language, he collects information about Mechatronics Engineering and communicates with his colleagues. ("European Language Portfolio Global Scale", Level B1) |
-
|
-
|
-
|
-
|
-
|
|
12 |
To be able to use the second foreign language at intermediate level. |
-
|
-
|
-
|
-
|
-
|
|
13 |
To recognize the need for lifelong learning; to be able to access information; to be able to follow developments in science and technology; to be able to relate the knowledge accumulated throughout the human history to Mechatronics Engineering. |
-
|
-
|
-
|
-
|
-
|
*1 Lowest, 2 Low, 3 Average, 4 High, 5 Highest
An autonomous robot that can re-route using artificial intelligence, when it encounters an obstacle, has been developed with the project ...
As Izmir University of Economics transforms into a world-class university, it also raises successful young people with global competence.
More..Izmir University of Economics produces qualified knowledge and competent technologies.
More..Izmir University of Economics sees producing social benefit as its reason for existence.
More..